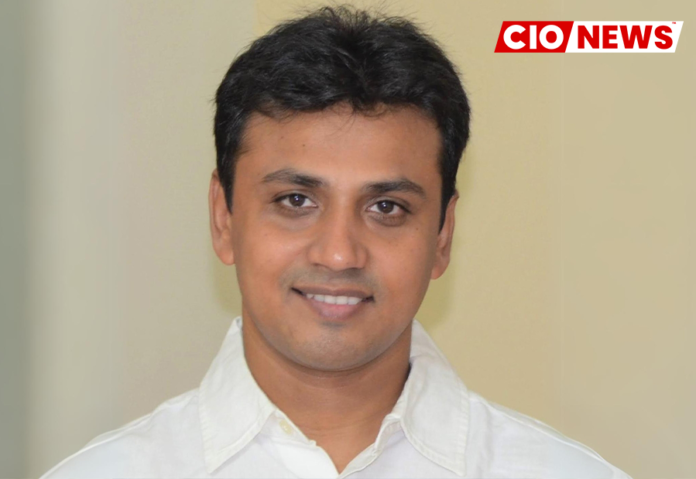
Trends Shaping the Data Streams from Collection to Action
Modern business practices have redefined the expectations placed on data processing and analytics. Organizations seek to have data that can guide strategic decision-making in real time and improve operational efficiency. Data now plays a key role in driving competitive advantage, customer experiences, and innovation.
At the same time, with a deluge of a variety of data from diverse sources, there is increased complexity in architecting data pipelines. Despite the challenges, data professionals are expected to provide a comprehensive and interconnected data view with agility and responsiveness.
Organizations are no longer content with static, retrospective insights derived from their traditional analytics practices. They expect immediate, actionable information that allows for swift decision-making in the ever-changing business environment. This necessitates a paradigm shift in data workflows, from conventional batch processing to the agility and immediacy offered by dynamic and unified data streams.
The turn of a new year is a good time to take a deeper look at what to expect in the near future in data’s journey from being a collection of facts to becoming real, actionable business intelligence:
Enhanced Connectivity and Integration
Effective data processing today hinges on the ability to seamlessly connect with diverse sources, may they be online platforms, cloud services, or business applications. Integration tools are not merely about establishing links; they are expected to orchestrate the data flows as per business needs. Workflows must be streamlined, automating the Extract, Transform, and Load (ETL) processes with capabilities to handle batch, micro-batch, and streaming data at scale.
The shift to cloud-based solutions stands out as another definitive trend. Cloud platforms offer scalability, flexibility, cost-effectiveness, and enhanced security. Leveraging cloud-native services like serverless computing, AI, and ML tools, organizations can automate and optimize data integration tasks. Cloud-based data processing also facilitates seamless collaboration and ubiquitous data accessibility for teams and stakeholders globally.
The integration of AI and machine learning makes data pipelining simpler and faster for users, further refining and enhancing the integration process. Complementing this is the trend of self-service data integration, which empowers business users to construct and manage their data pipelines without coding or technical expertise.
It is vital to build connectivity solutions that are not static but adaptable. This ensures that connectivity infrastructures can seamlessly accommodate emerging data sources, technologies, and industry trends, providing a foundation for sustained relevance in the future.
Real-time ETL for Insights On-the-Go
The conventional approach to processing time-stamped data in batch mode has its inherent challenges. It is no longer sufficient in industries where real-time insights are crucial for operational and strategic decisions.
To make it possible, real-time ETL has emerged as a critical component within data streams, enabling the instantaneous readiness of data to be fed into downstream analytics or BI applications. This capability is vital for businesses requiring prompt, data-driven decision-making.
Real-time ETL in data streams has become a strategic imperative for businesses characterized by rapid change and fierce competition. The ability to process data events as they happen provides a distinct competitive edge. From financial services responding to market fluctuations to bidding and targeting on programmatic advertising platforms, real-time insights empower organizations to make informed decisions to stay ahead in the race.
Data Catalogs
A data catalog serves as a comprehensive inventory and an index to organize diverse datasets within an organization. Beyond mere listing, data catalogs encapsulate metadata, schema information, and contextual details. It provides a map for data professionals to navigate the complex terrains of structured and unstructured data, which is especially vital with the escalating volume and complexity of data.
Metadata not only includes the basic attributes of the data but also contextual information, usage guidelines, and lineage details. It enhances data discoverability, unlocking its full potential as well as ensuring data quality.
Data catalogs also break down silos and enable a wider audience, including non-technical users, to discover and comprehend the data, facilitating data democratization.
Data Visualization
Visualization tools go beyond presenting numbers; they craft visual narratives that convey complex information in an accessible manner. By leveraging the power of visuals, insights are communicated and understood more effectively, enabling stakeholders to grasp the implications of the data without getting overwhelmed with tabular data.
Visualizations like charts, graphs, and dashboards provide clarity. Visualization tools have a user-centric design, ensuring that the visuals are not just informative but also intuitive. Drag-and-drop interfaces, customizable dashboards, and interactive elements allowing changing parameters empower users to explore data without technical dependence. This helps them uncover nuances and patterns in a self-service manner.
Hybrid Cloud is Here to Stay
Hybrid cloud is not just a fad; it will continue to be a fixture in data pipelines in the near future. It balances practicality and efficiency, taking the best out of legacy and contemporary IT strategies.
A hybrid architecture of on-premises infrastructure and cloud services helps it retain the familiarity and control of its own on-site systems while still allowing it to harness the cloud’s benefits. Businesses can scale up or down, innovate, and be agile without upheaving their entire infrastructure. Most importantly, it accommodates the security concerns of handling sensitive data on-premise.
Hybrid Cloud will persist in being useful as it remains adaptable, balanced, and efficient.
Data in Motion for Real-Time Processing
Data streaming continues to mature with innovative solutions, emerging as an essential backbone for business operations and decision support. There is a rise in demand for reliable and immediate data and event delivery to support applications. More and more organizations are choosing a data-streaming-native approach for their analytics and BI applications.
Consider ride-hailing apps that are built on streaming data. The platforms orchestrate several data points, like driver locations, traffic, dynamic pricing, etc., to provide a seamless experience for the user. Real-time data streaming ensures dynamic adjustments to provide accurate arrival and pricing estimates, match drivers with riders efficiently, and make payment updates. The continuous flow of data into the micro-services within the application is the lifeline that provides a superior level of customer satisfaction and repeat business, leading to business success.
Enterprises expect to leverage data differently today, asking for real-time insights for immediate decision-making. There is a realization that streaming data is a force multiplier when it comes to moving, analyzing, and sharing data. In the future, new use cases and products will continue to arise that are based on streaming data architectures.
Also read: The Road Ahead: Predictions for the Future Evolution of Artificial Intelligence
Do Follow: CIO News LinkedIn Account | CIO News Facebook | CIO News Youtube | CIO News Twitter
About us:
CIO News, a proprietary of Mercadeo, produces award-winning content and resources for IT leaders across any industry through print articles and recorded video interviews on topics in the technology sector such as Digital Transformation, Artificial Intelligence (AI), Machine Learning (ML), Cloud, Robotics, Cyber-security, Data, Analytics, SOC, SASE, among other technology topics.